How Generative AI Is Changing Creative Work
Large language and image AI models, occasionally called generative AI or foundation models, have created a new set of openings for businesses and professionals that perform content creation. Some of these openings include.
How Generative AI Is Changing Creative Work
Large language and image AI models, occasionally called generative AI or foundation models, have created a new set of openings for businesses and professionals that perform content creation. Some of these openings include.
- Automated happy generation Large language and image AI models can be used to automatically induce content, similar as papers, blog posts, or social media posts. This can be a precious time- saving tool for businesses and professionals who produce content on a regular basis.
- Advanced content quality AI-generated content can be of advanced quality than content created by humans, due to the fact that AI models are suitable to learn from a large quantum of data and identify patterns that humans may not be suitable to see. This can affect more accurate and instructional content.
- Increased content variety AI models can induce a variety of content types, including textbook, images, and videotape. This can help businesses and professionals to produce further different and intriguing content that appeals to a wider range of people.
- Individualized content AI models can induce substantiated content grounded on the preferences of individual druggies. This can help businesses and professionals to produce content that's more likely to be of interest to their target followership, and thus more likely to be read or participated.
How complete is this technology at mimicking mortal sweats at creative work? Well, for an illustration, the accentuated textbook above was written by GPT- 3, a “ large language model ”( LLM) created by OpenAI, in response to the first judgment , which we wrote. GPT- 3’s textbook reflects the strengths and sins of utmost AI- generated content.
- Automated happy generation Large language and image AI models can be used to automatically induce content, similar as papers, blog posts, or social media posts. This can be a precious time- saving tool for businesses and professionals who produce content on a regular basis.
- Advanced content quality AI-generated content can be of advanced quality than content created by humans, due to the fact that AI models are suitable to learn from a large quantum of data and identify patterns that humans may not be suitable to see. This can affect more accurate and instructional content.
- Increased content variety AI models can induce a variety of content types, including textbook, images, and videotape. This can help businesses and professionals to produce further different and intriguing content that appeals to a wider range of people.
- Individualized content AI models can induce substantiated content grounded on the preferences of individual druggies. This can help businesses and professionals to produce content that's more likely to be of interest to their target followership, and thus more likely to be read or participated.
How complete is this technology at mimicking mortal sweats at creative work? Well, for an illustration, the accentuated textbook above was written by GPT- 3, a “ large language model ”( LLM) created by OpenAI, in response to the first judgment , which we wrote. GPT- 3’s textbook reflects the strengths and sins of utmost AI- generated content.
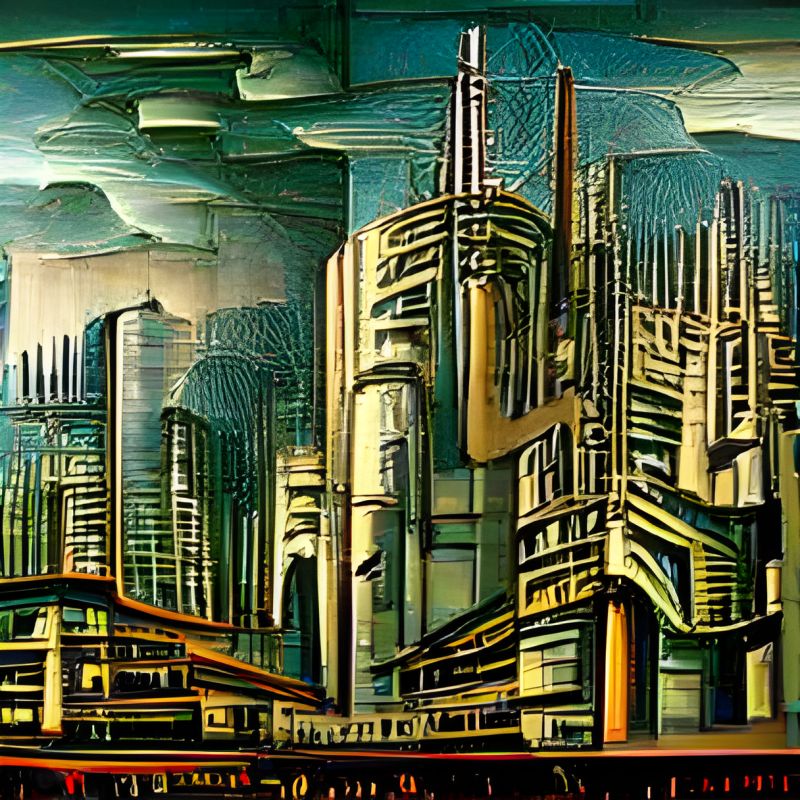
First, it's sensitive to the prompts fed into it; we tried several volition prompts before settling on that judgment. Second, the system writes nicely well; there are no grammatical miscalculations, and the word choice is applicable. Third, it would profit from editing; we'd not typically begin a composition like this bone with a numbered list, for illustration. Eventually, it came up with ideas that we did n’t suppose of. The last point about substantiated content, for illustration, isn't one we'd have considered.
Overall, it provides a good illustration of the implicit value of these AI models for businesses. They hang to upend the world of content creation, with substantial impacts on marketing, software, design, entertainment, and interpersonal dispatches. This isn't the “ artificial general intelligence ” that humans have long pictured and stressed, but it may look that way to casual spectators.
What Is Generative AI?
Generative AI can formerly do a lot. It’s suitable to produce textbook and images, gauging blog posts, program law, poetry, and artwork( and indeed winning competitions, controversially). The software uses complex machine literacy models to prognosticate the coming word grounded on former word sequences, or the coming image grounded on words describing former images. LLMs began at Google Brain in 2017, where they were originally used for restatement of words while conserving the environment. Since also, large language and textbook- to- image models have mushroomed at leading tech enterprises including Google( BERT and LaMDA), Facebook( OPT- 175B, BlenderBot), and OpenAI, a nonprofit in which Microsoft is the dominant investor( GPT- 3 for textbook, DALL- E2 for images, and Whisper for speech). Online communities similar as Midjourney( which helped win the art competition), and open- source providers like HuggingFace, have also created generative models.
These models have largely been confined to major tech companies because training them requires massive quantities of data and calculating power. GPT- 3, for illustration, was originally trained on 45 terabytes of data and employs 175 billion parameters or portions to make its prognostications; a single training run for GPT- 3 bring$ 12 million. Wu Dao2.0, a Chinese model, has1.75 trillion parameters. Most companies do n’t have the data center capabilities or calculating budgets to train their own models of this type from scrape.
But once a generative model is trained, it can be “fine-tuned” for a particular content sphere with much lower data. This has led to technical models of BERT — for biomedical content( BioBERT), legal content( Legal- BERT), and French textbook( CamemBERT) — and GPT- 3 for a wide variety of specific purposes. NVIDIA’s BioNeMo is a frame for training, structure and planting large language models at supercomputing scale for generative chemistry, proteomics, and DNA/RNA. OpenAI has set up that as many as 100 specific exemplifications of sphere-specific data can mainly ameliorate the delicacy and applicability of GPT- 3’s labors.
To use generative AI effectively, you still need mortal involvement at both the morning and the end of the process.
To start with, a human must enter an advice into a generative model in order to have it produce content. Generally speaking, creative prompts yield creative labors. “ Prompt mastermind ” is likely to become an established profession, at least until the coming generation of indeed smarter AI emerges. The field has formerly led to an 82- runner book of DALL- E 2 image prompts, and a prompt business in which for a small figure bone can buy other druggies ’ prompts. The utmost druggies of these systems will need to try several different prompts before achieving the asked outgrowth.
Also, once a model generates content, it'll need to be estimated and edited precisely by a mortal. Indispensable prompt labors may be combined into a single document. Image generation may bear substantial manipulation. Jason Allen, who won the Colorado “ digitally manipulated photography ” contest with help from Midjourney, told a journalist that he spent more than 80 hours making more than 900 performances of the art, and fine- tuned his prompts over and over. He also bettered the outgrowth with Adobe Photoshop, increased the image quality and sharpness with another AI tool, and published three pieces on oil.
Generative AI models are incredibly different. They can take in similar content as images, longer textbook formats, emails, social media content, voice recordings, program law, and structured data. They can deal with new content, restatements, answers to questions, sentiment analysis, summaries, and indeed vids. These universal content machines have numerous implicit operations in business, several of which we describe below.
First, it's sensitive to the prompts fed into it; we tried several volition prompts before settling on that judgment. Second, the system writes nicely well; there are no grammatical miscalculations, and the word choice is applicable. Third, it would profit from editing; we'd not typically begin a composition like this bone with a numbered list, for illustration. Eventually, it came up with ideas that we did n’t suppose of. The last point about substantiated content, for illustration, isn't one we'd have considered.
Overall, it provides a good illustration of the implicit value of these AI models for businesses. They hang to upend the world of content creation, with substantial impacts on marketing, software, design, entertainment, and interpersonal dispatches. This isn't the “ artificial general intelligence ” that humans have long pictured and stressed, but it may look that way to casual spectators.
What Is Generative AI?
Generative AI can formerly do a lot. It’s suitable to produce textbook and images, gauging blog posts, program law, poetry, and artwork( and indeed winning competitions, controversially). The software uses complex machine literacy models to prognosticate the coming word grounded on former word sequences, or the coming image grounded on words describing former images. LLMs began at Google Brain in 2017, where they were originally used for restatement of words while conserving the environment. Since also, large language and textbook- to- image models have mushroomed at leading tech enterprises including Google( BERT and LaMDA), Facebook( OPT- 175B, BlenderBot), and OpenAI, a nonprofit in which Microsoft is the dominant investor( GPT- 3 for textbook, DALL- E2 for images, and Whisper for speech). Online communities similar as Midjourney( which helped win the art competition), and open- source providers like HuggingFace, have also created generative models.
These models have largely been confined to major tech companies because training them requires massive quantities of data and calculating power. GPT- 3, for illustration, was originally trained on 45 terabytes of data and employs 175 billion parameters or portions to make its prognostications; a single training run for GPT- 3 bring$ 12 million. Wu Dao2.0, a Chinese model, has1.75 trillion parameters. Most companies do n’t have the data center capabilities or calculating budgets to train their own models of this type from scrape.
But once a generative model is trained, it can be “fine-tuned” for a particular content sphere with much lower data. This has led to technical models of BERT — for biomedical content( BioBERT), legal content( Legal- BERT), and French textbook( CamemBERT) — and GPT- 3 for a wide variety of specific purposes. NVIDIA’s BioNeMo is a frame for training, structure and planting large language models at supercomputing scale for generative chemistry, proteomics, and DNA/RNA. OpenAI has set up that as many as 100 specific exemplifications of sphere-specific data can mainly ameliorate the delicacy and applicability of GPT- 3’s labors.
To use generative AI effectively, you still need mortal involvement at both the morning and the end of the process.
To start with, a human must enter an advice into a generative model in order to have it produce content. Generally speaking, creative prompts yield creative labors. “ Prompt mastermind ” is likely to become an established profession, at least until the coming generation of indeed smarter AI emerges. The field has formerly led to an 82- runner book of DALL- E 2 image prompts, and a prompt business in which for a small figure bone can buy other druggies ’ prompts. The utmost druggies of these systems will need to try several different prompts before achieving the asked outgrowth.
Also, once a model generates content, it'll need to be estimated and edited precisely by a mortal. Indispensable prompt labors may be combined into a single document. Image generation may bear substantial manipulation. Jason Allen, who won the Colorado “ digitally manipulated photography ” contest with help from Midjourney, told a journalist that he spent more than 80 hours making more than 900 performances of the art, and fine- tuned his prompts over and over. He also bettered the outgrowth with Adobe Photoshop, increased the image quality and sharpness with another AI tool, and published three pieces on oil.
Generative AI models are incredibly different. They can take in similar content as images, longer textbook formats, emails, social media content, voice recordings, program law, and structured data. They can deal with new content, restatements, answers to questions, sentiment analysis, summaries, and indeed vids. These universal content machines have numerous implicit operations in business, several of which we describe below.
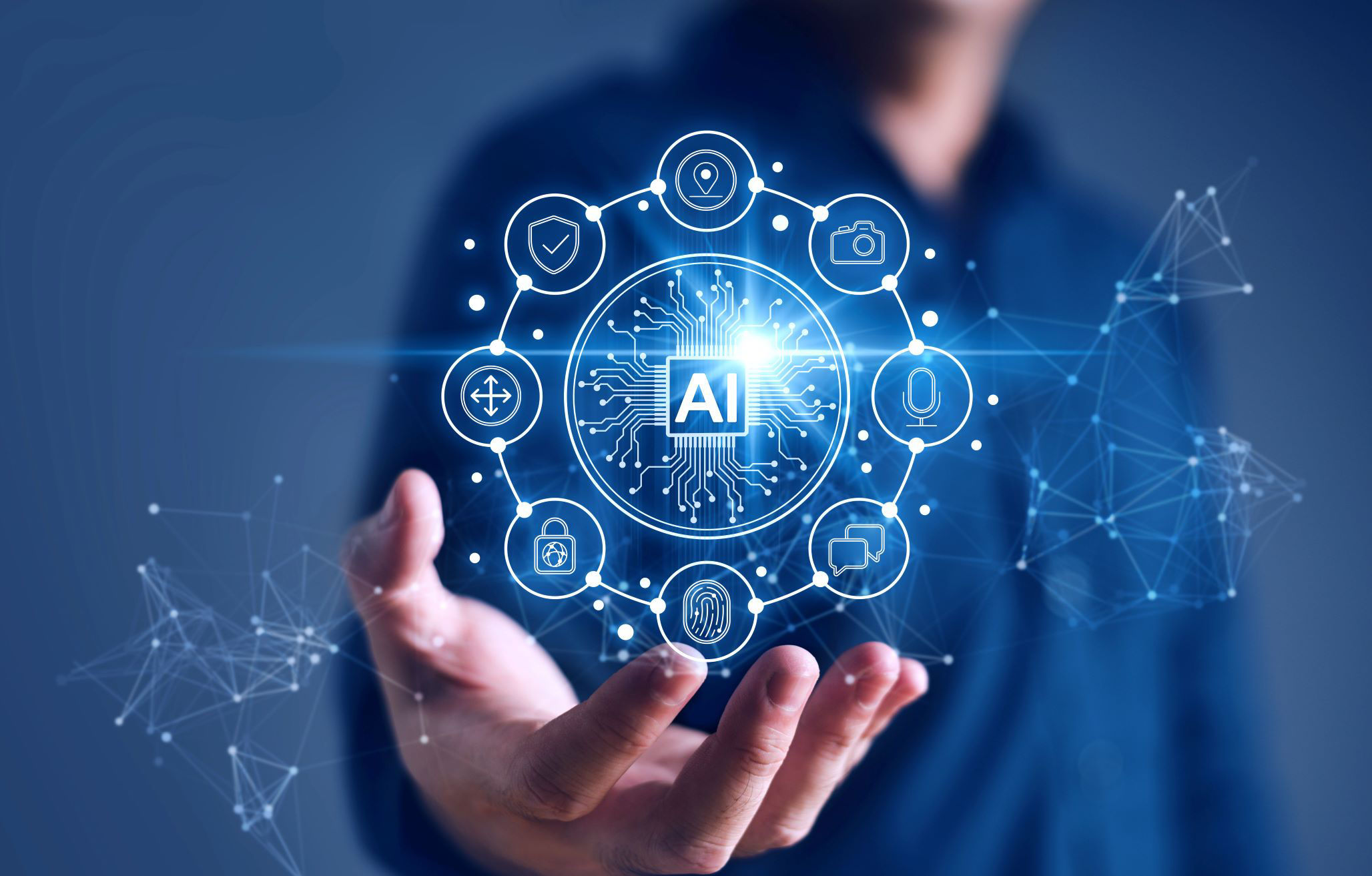
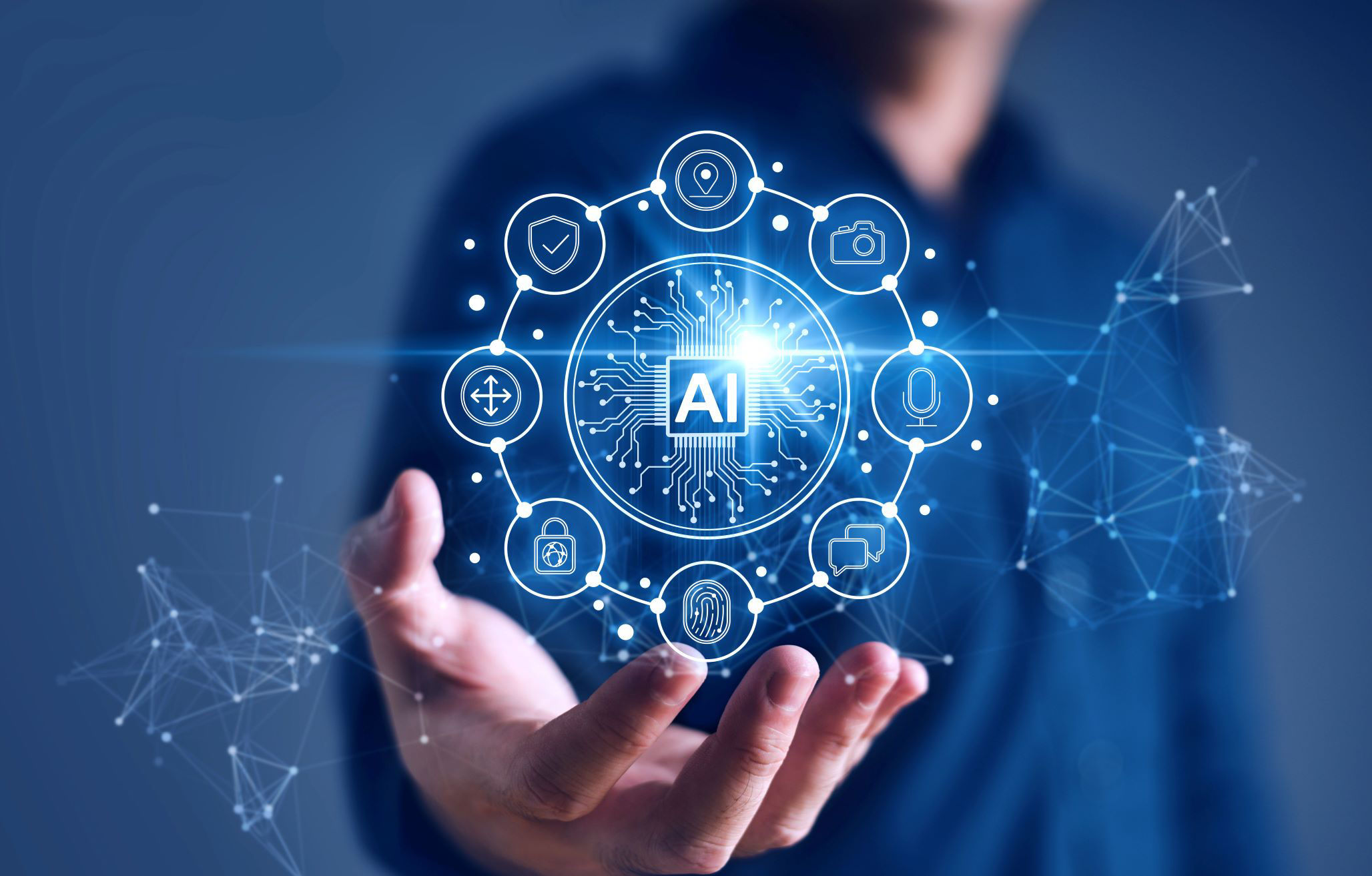
Marketing operations
These generative models are potentially precious across a number of business functions, but marketing operations are maybe the most common. Jasper, for illustration, a marketing- concentrated interpretation of GPT- 3, can produce blogs, social media posts, web dupe, deals emails, advertisements, and other types of client- facing content. It maintains that it constantly tests its labors with A/ B testing and that its content is optimized for hunt machine placement. Jasper also fine tunes GPT- 3 models with their guests stylish labors, which Jasper’s directors say has led to substantial advancements. The utmost of Jasper’s guests are individuals and small businesses, but some groups within larger companies also make use of its capabilities. At the pall calculating company VMWare, for illustration, pens use Jasper as they induce original content for marketing, from dispatch to product juggernauts to social media dupe.
Marketing operations
These generative models are potentially precious across a number of business functions, but marketing operations are maybe the most common. Jasper, for illustration, a marketing- concentrated interpretation of GPT- 3, can produce blogs, social media posts, web dupe, deals emails, advertisements, and other types of client- facing content. It maintains that it constantly tests its labors with A/ B testing and that its content is optimized for hunt machine placement. Jasper also fine tunes GPT- 3 models with their guests stylish labors, which Jasper’s directors say has led to substantial advancements. The utmost of Jasper’s guests are individuals and small businesses, but some groups within larger companies also make use of its capabilities. At the pall calculating company VMWare, for illustration, pens use Jasper as they induce original content for marketing, from dispatch to product juggernauts to social media dupe.
Rosa Lear, director of product- led growth, said that Jasper helped the company ramp up our content strategy, and the pens now have time to do better exploration, creativity, and strategy.
Kris Ruby, the proprietor of public relations and social media agency Ruby Media Group, is now using both textbook and image generation from generative models. She says that they're effective at maximizing hunt machine optimization( SEO), and in PR, for substantiated pitches to pens. These new tools, she believes, open up a new frontier in brand challenges, and she helps to produce AI programs for her guests. When she uses the tools, she says, “The AI is 10, I'm 90” because there's so important egging, editing, and replication involved. She feels that these tools make one’s jotting more and more complete for hunting machine discovery, and that image generation tools may replace the request for stock prints and lead to a belle epoque of creative work.
DALL- E 2 and other image generation tools are formerly being used for advertising. Heinz, for illustration, used an image of a ketchup bottle with a marker analogous to Heinz’s to argue that “This is what ‘ketchup’ looks like to AI.” Of course, it meant only that the model was trained on a fairly large number of Heinz ketchup bottle prints. Nestle used an AI- enhanced interpretation of a Vermeer oil to help sell one of its yogurt brands. Sew Fix, the apparel company that formerly uses AI to recommend specific apparel to guests, is experimenting with DALL- E 2 to produce visualizations of apparel grounded on requested client preferences for color, fabric, and style. Mattel is using the technology to induce images for toy design and marketing.
Code Generation Applications
GPT- 3 in particular has also proven to be an effective, if not perfect, creator of computer program law. Given a description of a “grain” or small program function, GPT- 3’s Codex program — specifically trained for law generation — can produce law in a variety of different languages. Microsoft’s Github also has an interpretation of GPT- 3 for law generation called CoPilot. The newest performances of Codex can now identify bugs and fix miscalculations in its own law — and indeed explain what the law does — at least some of the time. The expressed goal of Microsoft isn't to exclude mortal programmers, but to make tools like Codex or CoPilot “brace programmers” with humans to ameliorate their speed and effectiveness.
The agreement on LLM- grounded law generation is that it works well for similar particles, although the integration of them into a larger program and the integration of the program into a particular specialized terrain still bear mortal programming capabilities. Deloitte has experimented considerably with Codex over the past several months, and has set up it to increase productivity for educated inventors and to produce some programming capabilities for those with no experience.
In a six- week airman at Deloitte with 55 inventors for 6 weeks, a maturity of druggies rated the performing law’s delicacy at 65 or better, with a maturity of the law coming from Codex. Overall, the Deloitte trial set up a 20 enhancement in law development speed for applicable systems. Deloitte has also used Codex to restate law from one language to another. The establishment’s conclusion was that it would still need professional inventors for the foreseeable future, but the increased productivity might bear less of them. As with other types of generative AI tools, they set up the better the prompt, the better the affair law.
Conversational operations
LLMs are decreasingly being used at the core of conversational AI or chatbots. They potentially offer lesser situations of understanding of discussion and environment mindfulness than current conversational technologies. Facebook’s BlenderBot, for illustration, which was designed for dialogue, can carry on long exchanges with humans while maintaining the environment. Google’s BERT is used to understand hunt queries, and is also an element of the company’s DialogFlow chatbot machine. Google’s LaMBA, another LLM, was also designed for dialog, and exchanges with it induced one of the company’s masterminds that it was a sentient being — an emotional feat, given that it’s simply prognosticating words used in discussion grounded on once exchanges.
None of these LLMs is a perfect prattler. They're trained on once mortal content and have a tendency to replicate any supremacist, sexist, or prejudiced language to which they were exposed in training. Although the companies that created these systems are working on filtering out hate speech, they've not yet been completely successful.
Knowledge Management Applications
One arising operation of LLMs is to employ them as a means of managing textbook- grounded( or potentially image or videotape- grounded) knowledge within an association. The labor intensiveness involved in creating structured knowledge bases has made large- scale knowledge operations delicate for numerous large companies. Still, some exploration has suggested that LLMs can be effective at managing an association’s knowledge when model training is fine- tuned on a specific body of textbook- grounded knowledge within the association. The knowledge within an LLM could be penetrated by questions issued as prompts."
Some companies are exploring the idea of LLM- grounded knowledge operation in confluence with the leading providers of marketable LLMs. Morgan Stanley, for illustration, is working with OpenAI’s GPT- 3 to fine- tune training on wealth operation content, so that fiscal counsels can both search for knowledge within the establishment and produce acclimatized content for guests fluently. It seems likely that druggies of similar systems will need training or backing in creating effective prompts, and that the knowledge labors of the LLMs might still need editing or review before being applied. Assuming that similar issues are addressed, LLMs could revitalize the field of knowledge operation and allow it to gauge much more effectively.
Deepfakes and Other Legal/Ethical enterprises
We've formerly seen that these generative AI systems lead fleetly to a number of legal and ethical issues. “Deepfakes,” or images and videos that are created by AI and purport to be realistic but are not, have formerly arisen in media, entertainment, and politics. Yet, still, the creation of deepfakes needed a considerable quantum of calculating skill. Now, still, nearly anyone will be suitable to produce them. OpenAI has tried to control fake images by “watermarking” each DALL- E 2 image with a distinctive symbol. further controls are likely to be needed in the future, still — particularly as generative videotape creation becomes mainstream.
Generative AI also raises multitudinous questions about what constitutes original and personal content. Since the created textbook and images aren't exactly like any former content, the providers of these systems argue that they belong to their prompt generators. But they're easily secondary to the former textbook and images used to train the models. Dispensable to say, these technologies will give substantial work for intellectual property attorneys in the coming times.
From these many exemplifications of business operations, it should be clear that we're now only scratching the face of what generative AI can do for associations and the people within them. It may soon be standard practice, for illustration, for similar systems to craft most or all of our written or image- grounded content — to give first drafts of emails, letters, papers, computer programs, reports, blog posts, donations, vids, and so forth. No doubt that the development of similar capabilities would have dramatic and unlooked-for counter accusations for content power and intellectual property protection, but they're also likely to revise knowledge and creative work. Assuming that these AI models continue to progress as they've in the short time they have, we can hardly imagine all of the openings and counter accusations that they may engender.
Rosa Lear, director of product- led growth, said that Jasper helped the company ramp up our content strategy, and the pens now have time to do better exploration, creativity, and strategy.
Kris Ruby, the proprietor of public relations and social media agency Ruby Media Group, is now using both textbook and image generation from generative models. She says that they're effective at maximizing hunt machine optimization( SEO), and in PR, for substantiated pitches to pens. These new tools, she believes, open up a new frontier in brand challenges, and she helps to produce AI programs for her guests. When she uses the tools, she says, “The AI is 10, I'm 90” because there's so important egging, editing, and replication involved. She feels that these tools make one’s jotting more and more complete for hunting machine discovery, and that image generation tools may replace the request for stock prints and lead to a belle epoque of creative work.
DALL- E 2 and other image generation tools are formerly being used for advertising. Heinz, for illustration, used an image of a ketchup bottle with a marker analogous to Heinz’s to argue that “This is what ‘ketchup’ looks like to AI.” Of course, it meant only that the model was trained on a fairly large number of Heinz ketchup bottle prints. Nestle used an AI- enhanced interpretation of a Vermeer oil to help sell one of its yogurt brands. Sew Fix, the apparel company that formerly uses AI to recommend specific apparel to guests, is experimenting with DALL- E 2 to produce visualizations of apparel grounded on requested client preferences for color, fabric, and style. Mattel is using the technology to induce images for toy design and marketing.
Code Generation Applications
GPT- 3 in particular has also proven to be an effective, if not perfect, creator of computer program law. Given a description of a “grain” or small program function, GPT- 3’s Codex program — specifically trained for law generation — can produce law in a variety of different languages. Microsoft’s Github also has an interpretation of GPT- 3 for law generation called CoPilot. The newest performances of Codex can now identify bugs and fix miscalculations in its own law — and indeed explain what the law does — at least some of the time. The expressed goal of Microsoft isn't to exclude mortal programmers, but to make tools like Codex or CoPilot “brace programmers” with humans to ameliorate their speed and effectiveness.
The agreement on LLM- grounded law generation is that it works well for similar particles, although the integration of them into a larger program and the integration of the program into a particular specialized terrain still bear mortal programming capabilities. Deloitte has experimented considerably with Codex over the past several months, and has set up it to increase productivity for educated inventors and to produce some programming capabilities for those with no experience.
In a six- week airman at Deloitte with 55 inventors for 6 weeks, a maturity of druggies rated the performing law’s delicacy at 65 or better, with a maturity of the law coming from Codex. Overall, the Deloitte trial set up a 20 enhancement in law development speed for applicable systems. Deloitte has also used Codex to restate law from one language to another. The establishment’s conclusion was that it would still need professional inventors for the foreseeable future, but the increased productivity might bear less of them. As with other types of generative AI tools, they set up the better the prompt, the better the affair law.
Conversational operations
LLMs are decreasingly being used at the core of conversational AI or chatbots. They potentially offer lesser situations of understanding of discussion and environment mindfulness than current conversational technologies. Facebook’s BlenderBot, for illustration, which was designed for dialogue, can carry on long exchanges with humans while maintaining the environment. Google’s BERT is used to understand hunt queries, and is also an element of the company’s DialogFlow chatbot machine. Google’s LaMBA, another LLM, was also designed for dialog, and exchanges with it induced one of the company’s masterminds that it was a sentient being — an emotional feat, given that it’s simply prognosticating words used in discussion grounded on once exchanges.
None of these LLMs is a perfect prattler. They're trained on once mortal content and have a tendency to replicate any supremacist, sexist, or prejudiced language to which they were exposed in training. Although the companies that created these systems are working on filtering out hate speech, they've not yet been completely successful.
Knowledge Management Applications
One arising operation of LLMs is to employ them as a means of managing textbook- grounded( or potentially image or videotape- grounded) knowledge within an association. The labor intensiveness involved in creating structured knowledge bases has made large- scale knowledge operations delicate for numerous large companies. Still, some exploration has suggested that LLMs can be effective at managing an association’s knowledge when model training is fine- tuned on a specific body of textbook- grounded knowledge within the association. The knowledge within an LLM could be penetrated by questions issued as prompts."
Some companies are exploring the idea of LLM- grounded knowledge operation in confluence with the leading providers of marketable LLMs. Morgan Stanley, for illustration, is working with OpenAI’s GPT- 3 to fine- tune training on wealth operation content, so that fiscal counsels can both search for knowledge within the establishment and produce acclimatized content for guests fluently. It seems likely that druggies of similar systems will need training or backing in creating effective prompts, and that the knowledge labors of the LLMs might still need editing or review before being applied. Assuming that similar issues are addressed, LLMs could revitalize the field of knowledge operation and allow it to gauge much more effectively.
Deepfakes and Other Legal/Ethical enterprises
We've formerly seen that these generative AI systems lead fleetly to a number of legal and ethical issues. “Deepfakes,” or images and videos that are created by AI and purport to be realistic but are not, have formerly arisen in media, entertainment, and politics. Yet, still, the creation of deepfakes needed a considerable quantum of calculating skill. Now, still, nearly anyone will be suitable to produce them. OpenAI has tried to control fake images by “watermarking” each DALL- E 2 image with a distinctive symbol. further controls are likely to be needed in the future, still — particularly as generative videotape creation becomes mainstream.
Generative AI also raises multitudinous questions about what constitutes original and personal content. Since the created textbook and images aren't exactly like any former content, the providers of these systems argue that they belong to their prompt generators. But they're easily secondary to the former textbook and images used to train the models. Dispensable to say, these technologies will give substantial work for intellectual property attorneys in the coming times.
From these many exemplifications of business operations, it should be clear that we're now only scratching the face of what generative AI can do for associations and the people within them. It may soon be standard practice, for illustration, for similar systems to craft most or all of our written or image- grounded content — to give first drafts of emails, letters, papers, computer programs, reports, blog posts, donations, vids, and so forth. No doubt that the development of similar capabilities would have dramatic and unlooked-for counter accusations for content power and intellectual property protection, but they're also likely to revise knowledge and creative work. Assuming that these AI models continue to progress as they've in the short time they have, we can hardly imagine all of the openings and counter accusations that they may engender.